The Importance of Water Quality Monitoring
Water is the essence of life—over 60% of our bodies are made up of it! From quenching our thirst to growing food, powering industries, and providing recreational fun, clean water is crucial to nearly every aspect of our lives. By monitoring its quality, we can safeguard our rivers, lakes, ponds, and streams from pollution, ensuring they remain healthy and vibrant [1].
Monitoring water quality, especially for harmful algal blooms (HABs or CyanoHABs), is more challenging today due to the many chemicals, fertilizers, sewage, and waste runoff into lakes, rivers, or oceans. With over 80,000 chemicals in industry use in the U.S. alone and worldwide HAB events, only a tiny fraction can be effectively analyzed, making it difficult to assess risks and fully manage contamination in our waters. [2], [3]. Current practical water quality monitoring processes are expensive, time-consuming, and labor-intensive. Among these challenges, repair and maintenance costs, human resources, manual processes, data quality, and efficiency are significant concerns that complicate effective and sustainable water monitoring [4], [5]. Figure 1 shows our drone sampling during a harmful algal bloom (HAB) event in August 2023 at Shelburne Pond, Vermont, USA.
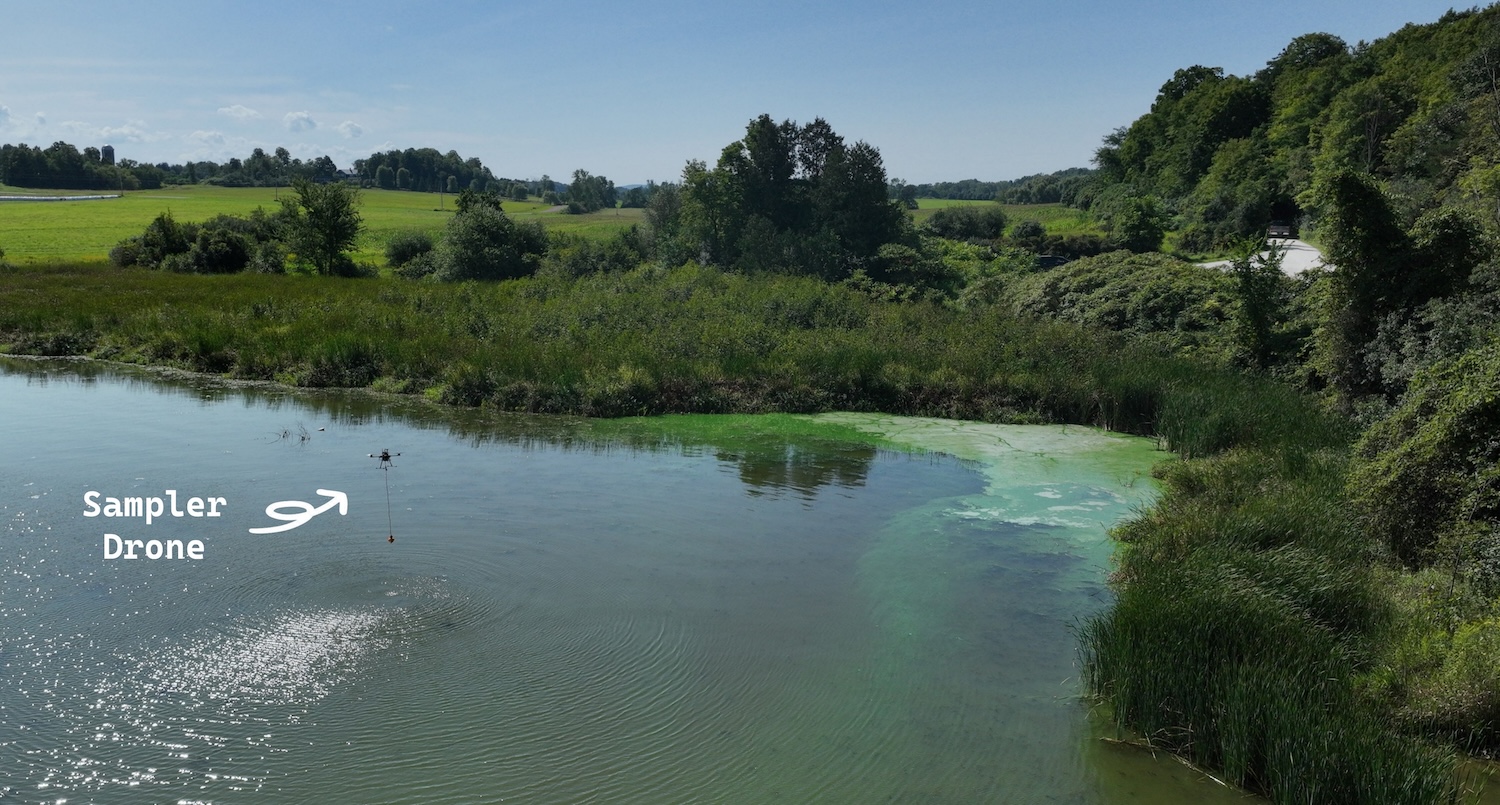
Figure 1. Sampling a HAB event, Shelburne Pond, Vermont, USA. Drone Operator: Soheyl Faghir Hagh, Photo by: Lauren Cresanti
Innovations in Water Sampling with Drones
Visual, taste, and odor changes alone cannot accurately assess water quality, particularly during HABs. Therefore, precise sensors and samplers are essential to make sure we can detect and capture these events promptly. Figure 2 shows our uncrewed aerial vehicle (UAV) system that can collect four samples (100 ml each) from four locations in a water body. This drone has an apparatus connected to our designed circuit board with wireless technology. This apparatus has four electromechanical samplers and sensors: temperature, pH, turbidity, and total dissolved solids (TDS). Each time the device is deployed in the water, the circuit senses the water, activates the samplers, reads the sensor data, and transmits this data over long distances (up to 1.6 km) through Long-Range (LoRa) technology. With a second drone for near-infrared imaging, our calibrated system can collect samples, measure water quality parameters, and collect images from the water body to form a comprehensive geographic information system (GIS) map. This map helps us understand the variation of HABs and potential mitigation strategies if necessary.
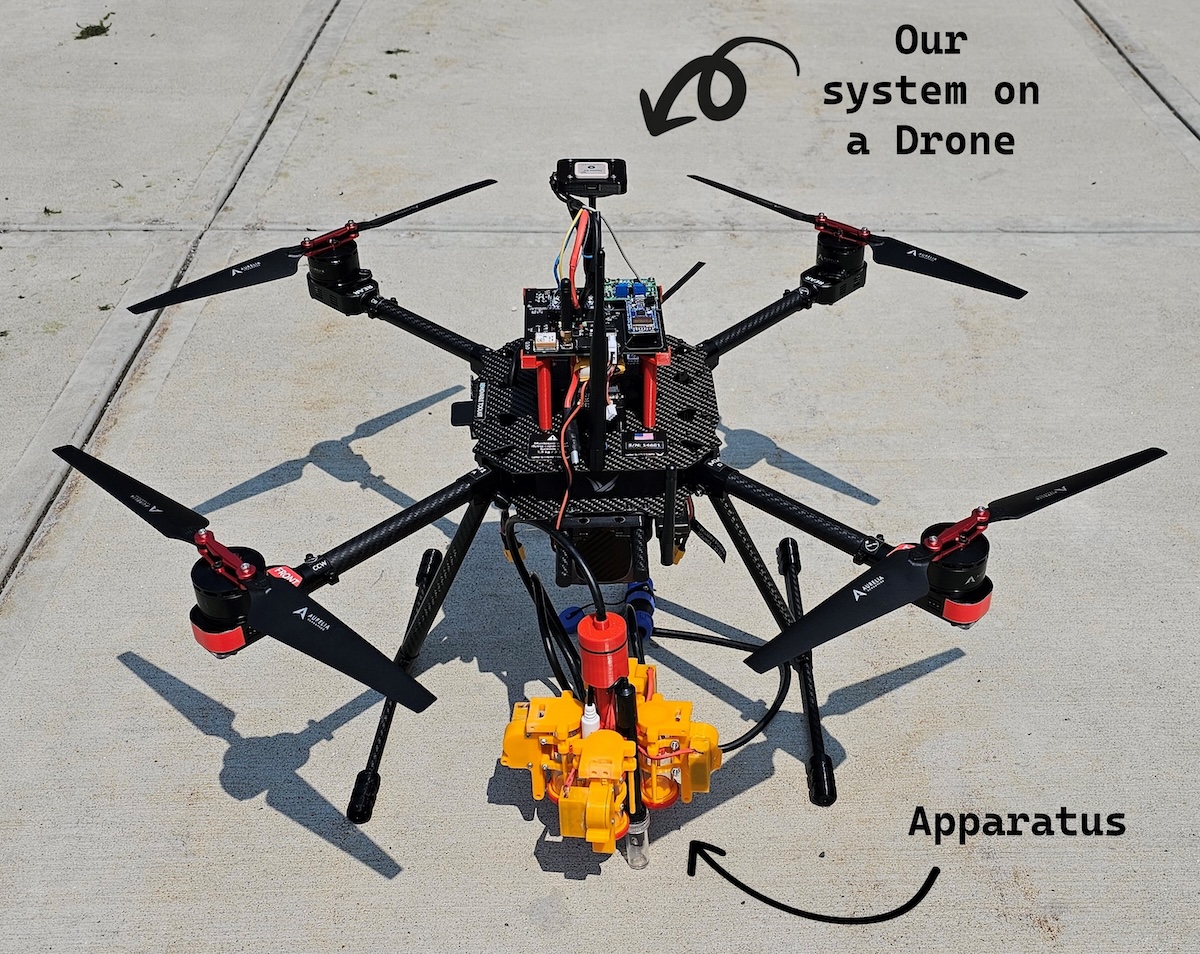
Figure 2. Our wireless sampler-sensor drone from [6], Credit: Soheyl Faghir Hagh
Our automated system’s advantage is eliminating the need for heavy equipment. Boats or kayaks are no longer needed! Furthermore, the total cost of our UAV system is lower than that of large autosamplers. It is possible to program the drone to fly to specific locations, collect samples, and return them to us at the launch site. The drone can fly for 1 hour with only 2 minutes for each sampling, so a large lake or pond can be covered thoroughly. It should be noted that this technology is novel in the sense that the end user can see the information in real-time. The drone broadcasts the encoded data, and the gateway receives this information. From there, it is relayed to the Web of Things for visualization and post-processing. The open-source design of this system allows for the integration of additional sensors, such as accurate fluorometers, spectrometers, or conductivity sensors.
Another significant advantage is that our system reduces the risk of human exposure to hazardous environments such as low-pH lakes. Hard-to-reach remote locations are easier to access, and water specialists, engineers, and technicians can collect samples multiple times daily. The full paper can be found here.
The use of Fluorometry and Nephelometry
Fluorometery, a sensitive analytical technique, detects the light emitted by a sample after it is excited. Nephelometry measures the amount of turbidity or cloudiness in a sample. We use these methods to detect CyanoHABs in water. It does not end there; our wireless system can also measure temperature. We detect Phycocyanin (PC) and Chlorophyll-a (Chl-a) fluorescence as proxies of Cyanobacteria and Phytoplankton, respectively. The system relies on the optical characteristics of PC and Chl-a, which emit light at distinct wavelengths when stimulated by an external light source. Chlorophyll-a emits light between 670-690 nm when incident light is in the 400-500 nm range, while phycocyanin emits around 650 nm when excited by light at approximately 600 nm [8]. Water cloudiness is measured in the near-infrared range.
Our system [7] pumps the fluid in a cuvette (with a peristaltic pump to minimize sample alteration) to make the measurements. It then excites the sample with three Light-Emitting Diodes (LEDs). The responses are measured using photodiodes as detectors. When light strikes the photodiodes, the photodiodes generate a tiny current, which we amplify and convert to electrical voltage to be measured. To minimize incidental light from LEDs, we use special glass filters in front of the photodiodes to remove it.
Each box must be meticulously calibrated in the laboratory with solutions for which the concentration of PC, Chl-a, and turbidity (also temperature) are known. So far, the calibration results indicate a promising result that agrees with commercial devices.
Finally, the microcontroller processes and prepares all the information for wireless transmission. We do the measurements every 15 minutes. Figure 3 shows a deployment example for our clarity box. The pipes and temperature probe are placed in the water, and the box is deployed for long-term monitoring. We are currently working on debugging our system to optimize it for field deployments, and soon, there will be many clarities on buoys monitoring the water for us. The open-access full paper is available here.
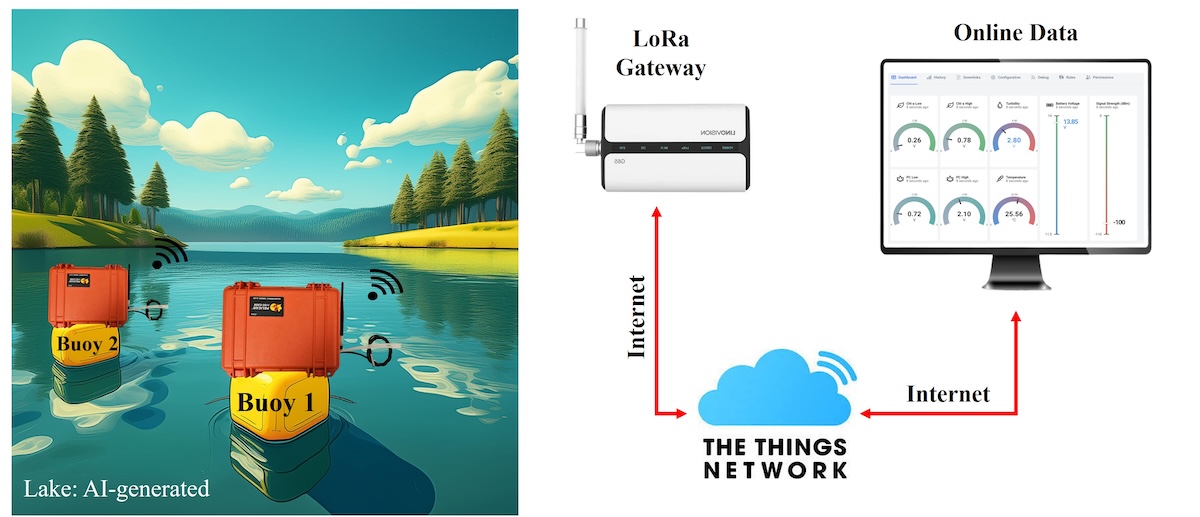
Figure 3. Our wireless fluorometer-nephelometer sensors (clarity) from [7], Credit: Soheyl Faghir Hagh
Summary
We have fabricated and calibrated two systems designed for a specific use. From the drone for quick and comprehensive sampling to the clarity box for long-term monitoring, both devices protect our water quality and inform the public should there be any risks to their health.
Copyright and permission:
The material presented here is with permission from IEEE for the drone work and under the Creators Common License for clarity.
References
[1] Water Quality Monitoring, Accessed August 2024. [Online.], Available: https://www.swfwmd.state.fl.us/residents/education/kids/water-quality-monitoring#:~:text=Each%20water%20body%20can%20contain,recognize%20and%20prevent%20contamination%20problems.
[2] D. N. Myers, “Why monitor water quality?” U.S. Geological Survey, Accessed August 2024. [Online.], Available: https://water.usgs.gov/owq/WhyMonitorWaterQuality.pdf
[3] “Harmful Algal Blooms (HABs) in Water Bodies,” Accessed July 2024. [Online.], Available: https://www.epa.gov/habs/what-are-effects-habs
[4] L. McDermand, “Overcome 5 Water Challenges When Monitoring Water,” Accessed August 2024. [Online.], Available: https://www.ysi.com/ysi-blog/water-blogged-blog/2019/02/how-to-overcome-5-water-quality-monitoring-challenges
[5] “Common Challenges Faced by Water Testing Laboratories,” Accessed August 2024. [Online.], Available: https://freelims.org/water-quality-testing-challenges-ways-to-overcome-them/
[6] S. F. Hagh et al., “Autonomous UAV-Mounted LoRaWAN System for Real-Time Monitoring of Harmful Algal Blooms (HABs) and Water Quality,” IEEE Sens. J., vol. 24, no. 7, pp. 11414–11424, 2024, doi: https://doi.org/10.1109/JSEN.2024.3364142.
[7] S. F. Hagh et al., “A Low-Cost LoRa Optical Fluorometer-Nephelometer for Wireless Monitoring of Water Quality Parameters in Real-Time,” IEEE Sens. J., p. 1, 2024, doi: https://doi.org/10.1109/JSEN.2024.3403416.
[8] A. Puiu, L. Fiorani, I. Menicucci, M. Pistilli, and A. Lai, “Submersible spectrofluorometer for real-time sensing of water quality,” Sensors (Switzerland), vol. 15, no. 6, pp. 14415–14434, 2015, doi: https://doi.org/10.3390/s150614415.
Author Information
Soheyl Faghir Hagh is a graduate research assistant at the Department of Electrical Engineering, University of Vermont, Burlington, Vermont. His research initiatives include developing LoRa wireless sensor nodes for environmental parameter monitoring, such as water quality and coastal environment. He is actively researching the LoRa mesh networks and sensors. His research interests include the Internet of Drones (IoD), LoRaWAN sensor nodes, machine learning, circuit design and fabrication, and device fabrication.
Related articles:
Dronelab to Showcase AI-Enhanced Pipeline Inspection Drones at Pipeline & Gas EXPO 2024